```{r echo = FALSE}
#| message: false
#| warning: false
pacman::p_load(tidyverse, readxl, knitr, kableExtra, performance, parameters,
latex2exp, see, patchwork, mfp, multcomp, emmeans, janitor, effectsize,
broom, ggmosaic, tinytable, ggrepel, glue, ggtext,
conflicted)
conflicts_prefer(dplyr::select)
conflicts_prefer(dplyr::filter)
cb_pal <- c("#000000", "#E69F00", "#56B4E9",
"#009E73", "#F0E442", "#F5C710",
"#0072B2", "#D55E00", "#CC79A7")
cbbPalette <- cb_pal
theme_marginal <- function() {
theme_minimal() +
theme(panel.grid.minor = element_blank(),
plot.background = element_rect(fill = "white", color = NA),
plot.title = element_text(size = 16, face = "bold"),
plot.subtitle = element_text(size = 12, face = "italic"),
plot.caption = element_text(face = "italic"),
axis.title = element_text(face = "bold"),
axis.text = element_text(size = 12),
strip.text = element_text(face = "bold"),
strip.background = element_rect(fill = "grey80", color = NA))
}
```
# Baustelle {#sec-construction}
*Letzte Änderung am `r format(fs::file_info("construction-zone.qmd")$modification_time, '%d. %B %Y um %H:%M:%S')`*
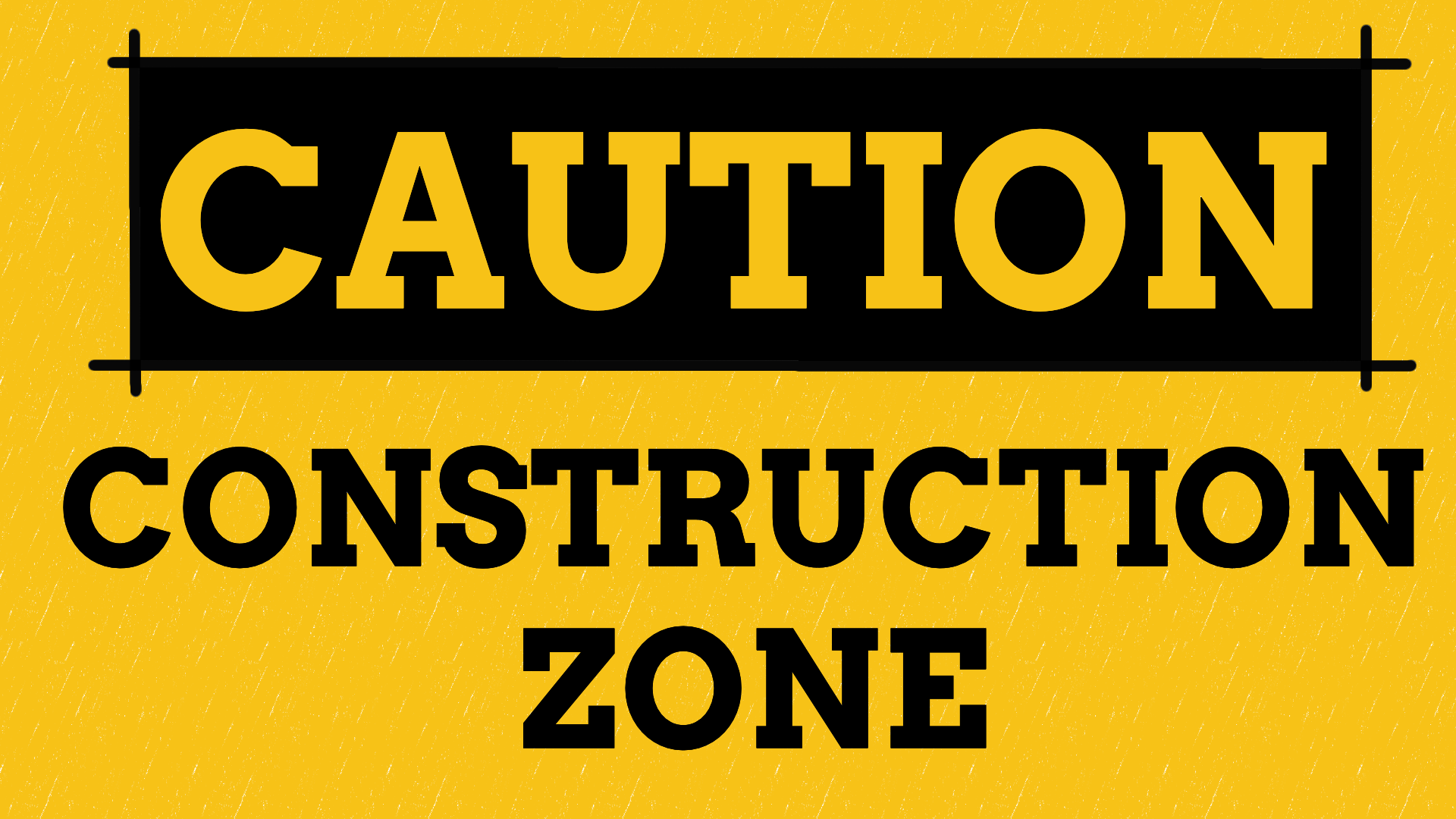 {fig-align="center" width="100%"}
::: {.callout-caution appearance="simple"}
## Stand des Kapitels: Konstruktion (seit 07.2025)
Dieses Kapitel wird in den nächsten Wochen geschrieben. Ich plane zum Beginn des WiSe 2025/26 eine neue Version des Kapitels erstellt zu haben. Während das Kapitel entsteht, funktioniert so manches dann nicht so wie es soll.
:::
::: {layout="[ 15,85 ] " layout-valign="top"}
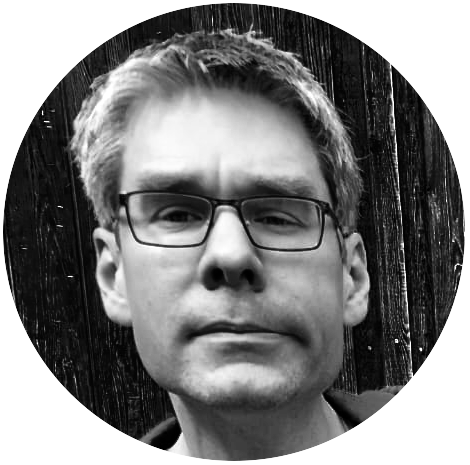 {fig-align="center" width="100%"}
> *"Aktuell wird gerade an den Marginal effects models gebaut... das wird jedenfalls so seine Zeit noch brauchen, bis ich hier einen Strang und Grund drin habe." --- Jochen Kruppa-Scheetz, meiner bescheidener Meinung nach.*
:::
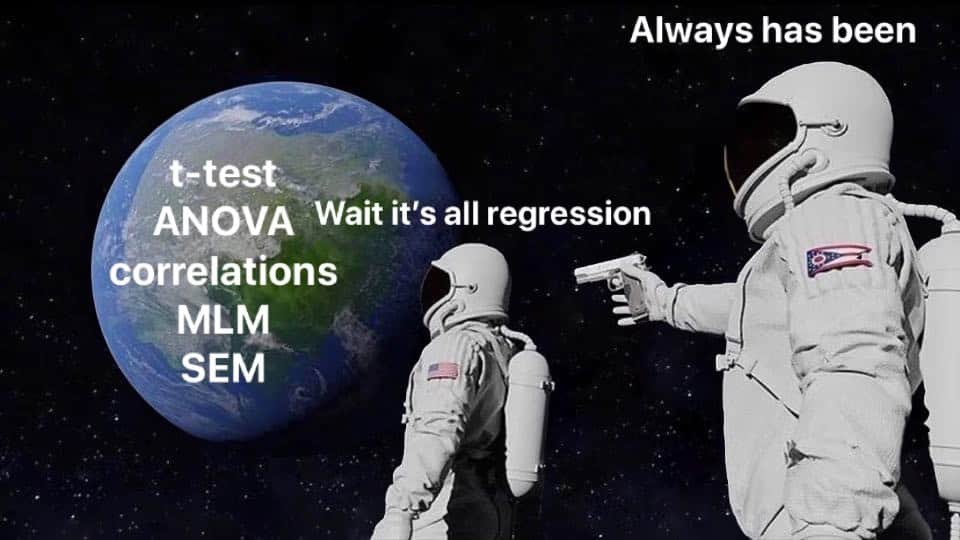 {#fig-all-regression fig-align="center" width="100%"}
1. Draw what you want to visualize
2. Make models for it
3. Select the best model
4. Visualize the best model
5. Investigate its parameters
## Genutzte R Pakete
Wir wollen folgende R Pakete in diesem Kapitel nutzen.
```{r echo = TRUE}
#| message: false
#| warning: false
pacman::p_load(tidyverse, gtsummary, marginaleffects, emmeans, scales,
ggpmisc, readxl, conflicted)
conflicts_prefer(dplyr::mutate)
conflicts_prefer(dplyr::summarize)
conflicts_prefer(dplyr::filter)
conflicts_prefer(ggplot2::annotate)
cb_pal <- c("#000000", "#E69F00", "#56B4E9",
"#009E73", "#F0E442", "#F5C710",
"#0072B2", "#D55E00", "#CC79A7")
##
nice_number <- label_number(style_negative = "minus", accuracy = 0.01)
nice_p <- label_pvalue(prefix = c("p < ", "p = ", "p > "))
find_intercept <- function(x1, y1, slope) {
intercept <- slope * (-x1) + y1
return(intercept)
}
```
An der Seite des Kapitels findest du den Link *Quellcode anzeigen*, über den du Zugang zum gesamten R-Code dieses Kapitels erhältst.
## Daten
```{r}
flea_model_tbl <- read_excel ("data/fleas_model_data.xlsx" ) |>
mutate (feeding = as_factor (feeding),
stage = as_factor (stage),
bonitur = as.numeric (bonitur),
infected = factor (infected, labels = c ("healthy" , "infected" ))) |>
select (feeding, stage, jump_length, weight, hatched, count_leg, bonitur, infected)
```
## Hypothesen
$$
Z=\frac{h(\hat{\theta})-H_0}{\sqrt{\hat{V}[ h(\hat{\theta}) ] }}
$$
```{r}
feeding_fit = lm (jump_length ~ 0 + feeding, data = flea_model_tbl)
coef (feeding_fit)
```
```{r}
summary (feeding_fit) |> coef ()
```
$$
Z = \cfrac{\hat{\beta_1}-H_0}{\sqrt{\widehat{V}[ \beta_1 ] }} = \cfrac{75.43938 - 0}{4.791469} = 15.74
$$
```{r}
2 * pt (15.74452 , df = 45 , lower.tail = FALSE )
```
```{r}
feeding_fit <- lm (jump_length ~ feeding * stage, data = flea_model_tbl)
coef (feeding_fit)
```
## Prädiktion
```{r}
simple_tbl <- flea_model_tbl |>
filter (stage == "adult" )
simple_fit <- lm (jump_length ~ feeding, simple_tbl)
coef (simple_fit)
```
```{r}
predictions (simple_fit)
avg_predictions (simple_fit)
```
```{r}
plot_predictions (simple_fit, by = "feeding" )
```
```{r}
simple_2_tbl <- flea_model_tbl |>
filter (stage == "adult" )
simple_2_fit <- lm (jump_length ~ weight* feeding, simple_2_tbl)
coef (simple_2_fit)
```
```{r}
#| eval: false
simple_2_fit |> broom:: augment ()
predictions (simple_2_fit)
avg_predictions (simple_2_fit, by = "feeding" )
tibble (weight = simple_2_tbl$ weight,
jump_length = simple_2_tbl$ jump_length,
feeding = simple_2_tbl$ feeding,
estimate = predictions (simple_2_fit)$ estimate) |>
ggplot (aes (weight, estimate, shape = feeding)) +
geom_point (aes (weight, jump_length), color = "blue" ) +
geom_point (color = "red" ) +
geom_line (aes (y = predict (simple_2_fit))) +
geom_hline (yintercept = avg_predictions (simple_2_fit, by = "feeding" )$ estimate)
```
```{r}
plot_predictions (simple_2_fit, by = c ("weight" , "feeding" ))
```
## Counterfactual
```{r}
avg_comparisons (feeding_fit,
by = "stage" ,
variables = list ("feeding" = "pairwise" ),
vcov = "HC3" )
```
## Weitere R Pakete
Das [ R Paket `{modelbased}` ](https://easystats.github.io/modelbased/)
## Marginal effects models
### Kontrafaktische Vergleiche
Kontrafaktische Vergleiche (eng. *counterfactual*)
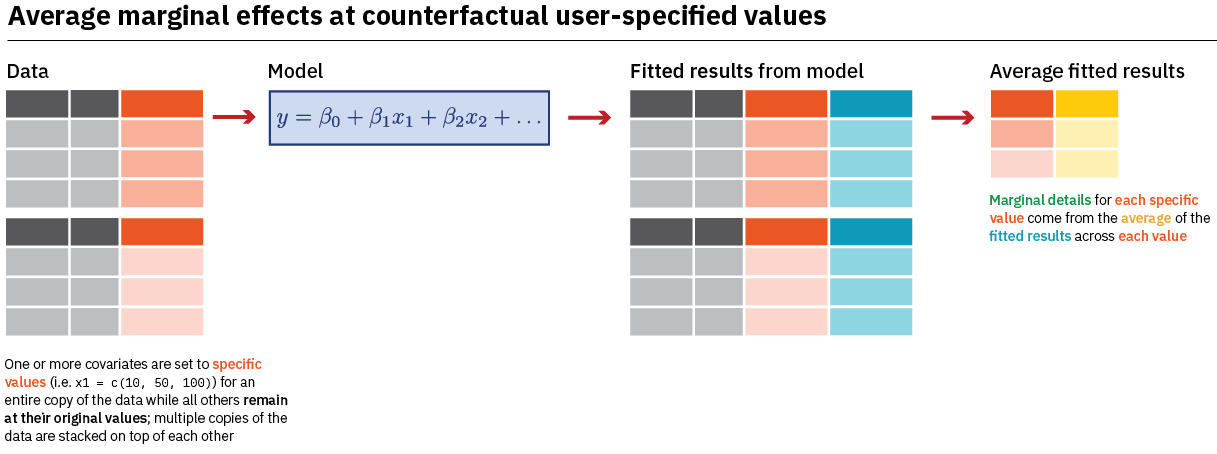 {#fig-utest-intro-04 fig-align="center" width="100%"}
```{r}
model_grp_sq <- lm (jump_length ~ weight* I (weight^ 2 )* feeding + count_leg,
data = flea_model_tbl)
tidy (model_grp_sq)
```
```{r}
cfct_data <- datagrid (model = model_grp_sq,
weight = c (5 , 15 ),
grid_type = "counterfactual" )
```
```{r}
nrow (flea_model_tbl)
nrow (cfct_data)
```
```{r}
mfx_cfct <- model_grp_sq |>
slopes (datagrid (weight = c (5 , 15 ),
grid_type = "counterfactual" ),
variables = "weight" )
rbind (head (mfx_cfct), tail (mfx_cfct))
```
```{r}
mfx_cfct |>
group_by (weight) |>
summarize (avg_slope = mean (estimate))
```
```{r}
model_grp_sq |>
avg_slopes (newdata = datagrid (weight = c (5 , 20 ),
grid_type = "counterfactual" ),
variables = "weight" ,
by = c ("weight" , "feeding" ))
```
## Analyse von Zeitreihen
Hier nochmal `{mgcv}` und `{gamm4}`
[ Introduction to Generalized Additive Mixed Models ](https://r.qcbs.ca/workshop08/book-en/introduction-to-generalized-additive-mixed-models-gamms.html)
`s()` und Interaktion mit `s(x_1, by = f_1)`
## Links
- [ Mixed ](https://stats.stackexchange.com/questions/95054/how-to-get-an-overall-p-value-and-effect-size-for-a-categorical-factor-in-a-mi)
- [ Mixed II ](https://bbolker.github.io/mixedmodels-misc/glmmFAQ.html)
- [ Marginal and conditional effects for GLMMs with {marginaleffects} \| Andrew Heiss – Andrew Heiss ](https://www.andrewheiss.com/blog/2022/11/29/conditional-marginal-marginaleffects/)
- [ R Paket `{gapminder}` ](https://github.com/jennybc/gapminder)
- [ Gapminder ](https://www.gapminder.org/data/)
- [ Lists are my secret weapon for reporting stats with knitr - Higher Order Functions ](https://tjmahr.github.io/lists-knitr-secret-weapon/)
- [ Visualizing {dplyr}’s mutate(), summarize(), group_by(), and ungroup() with animations \| Andrew Heiss – Andrew Heiss ](https://www.andrewheiss.com/blog/2024/04/04/group_by-summarize-ungroup-animations/)
## Referenzen {.unnumbered}